Kal Ramnarayan, Ph.D.
Co-founder President & Chief Scientific Officer, Sapient Discovery, San Diego, CA, USA
A cost-effective approach to Protein Structure-guided Drug Discovery: Aided by Bioinformatics, Chemoinformatics and computational chemistry
With the mapping of the human genome completed almost a decade ago, efforts are still underway to understand the gene products (i.e., proteins) in the human biological and disease pathways. Deciphering such information is very important for the discovery and development of small molecule drugs as well as protein therapeutics for various human diseases for which no cure exists. As an example, with more than 500 members, the kinase family of protein targets continues to be an important and attractive class for drug discovery. While how many of the members in this family are actually druggable is still to be established, there are several ongoing efforts on this class of proteins across a broad spectrum of disease categories. Even though in general the protein structural topology might looks similar, there are issues with respect selectivity of identified small molecule inhibitors when, the lead molecule discovery is carried out at the ATP binding site. As an added complexity, allosteric modulators are needed for some of the members, but the actual site for such modulation on the protein target can not resolved with uncertainty. In this presentation we will describe a bioinformatics and computational based platform for small molecule discovery for protein targets that are involved in protein-protein interactions as well as targets like kinases and phosphatases. We will describe a computational approach in which we have used an informatics based platform with several hundred kinases to sort through in silico and identify inhibitors that are likely to be highly selective in the lead generation phase. We will discuss the implication of this approach on the drug discovery of the kinase and phosphatase classes in general and independent of the disease category.
Srisairam Achuthan, Ph.D.
Senior Scientific Programmer, Research Informatics Division, Department of Information Sciences, City of Hope, CA, USA
Applying Machine learning for Automated Identification of Patient Cohorts
Srisairam Achuthan, Mike Chang, Ajay Shah, Joyce Niland
Patient cohorts for a clinical study are typically identified based on specific selection criteria. In most cases considerable time and effort are spent in finding the most relevant criteria that could potentially lead to a successful study. For complex diseases, this process can be more difficult and error prone since relevant features may not be easily identifiable. Additionally, the information captured in clinical notes is in non-coded text format. Our goal is to discover patterns within the coded and non-coded fields and thereby reveal complex relationships between clinical characteristics across different patients that would be difficult to accomplish manually. Towards this, we have applied machine learning techniques such as artificial neural networks and decision trees to determine patients sharing similar characteristics from available medical records. For this proof of concept study, we used coded and non-coded (i.e., clinical notes) patient data from a clinical database. Coded clinical information such as diagnoses, labs, medications and demographics recorded within the database were pooled together with non-coded information from clinical notes including, smoking status, life style (active / inactive) status derived from clinical notes. The non-coded textual information was identified and interpreted using a Natural Language Processing (NLP) tool I2E from Linguamatics.
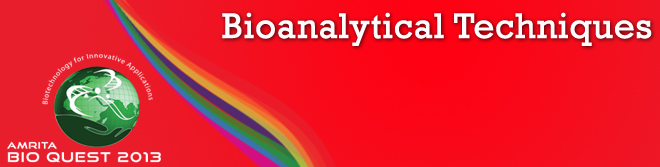
Tejaswini Subbannayya, Nandini A. Sahasrabuddhe, Arivusudar Marimuthu, Santosh Renuse, Gajanan Sathe, Srinivas M. Srikanth, Mustafa A. Barbhuiya, Bipin Nair, Juan Carlos Roa, Rafael Guerrero-Preston, H. C. Harsha, David Sidransky, Akhilesh Pandey, T. S. Keshava Prasad and Aditi Chatterjee
Proteomic profiling of gallbladder cancer secretome – a source for circulatory biomarker discovery
Gallbladder cancer (GBC) is the fifth most common cancer of the gastrointestinal tract and one of the common malignancies that occur in the biliary tract (Misra et al. 2006; Lazcano-Ponce et al. 2001). It has a poor prognosis with survival of less than 5 years in 90% of the cases (Misra et al. 2003). The etiology is ill-defined. Several risk factors have been reported including cholelithiasis, obesity, female gender and exposure to carcinogens (Eslick 2010; Kumar et al. 2006). Poor prognosis in GBC is mainly due to late presentation of the disease and lack of reliable biomarkers for early diagnosis. This emphasizes the need to identify and characterize cancer biomarkers to aid in the diagnosis and prognosis of GBC. Secreted proteins are an important class of molecules which can be detected in body fluids and has been targeted for biomarker discovery. There are challenges faced in the proteomic interrogation of body fluids especially plasma such as low abundance of tumor secreted proteins, high complexity and high abundance of other proteins that are not released by the tumor cells (Tonack et al. 2009). Profiling of conditioned media from the cancer cell lines can be used as an alternate means to identify secreted proteins from tumor cells (Kashyap et al. 2010; Marimuthu et al. 2012). We analyzed the invasive property of 7 GBC cell lines (SNU-308, G-415, GB-d1, TGBC2TKB, TGBC24TKB, OCUG-1 and NOZ). Four cell lines were selected for analysis of the cancer secretome based on the invasive property of the cells. We employed isobaric tags for relative and absolute quantitation (iTRAQ) labeling technology coupled with high resolution mass spectrometry to identify and characterize secretome from the panel of 4GBC cancer cells mentioned above. In total, we have identified around 2,000 proteins of which 175 were secreted at differential abundance across all the four cell lines. This secretome analysis will act as a reservoir of candidate biomarkers. Currently, we are investigating and validating these candidate markers from GBC cell secretome. Through this study, we have shown mass spectrometry-based quantitative proteomic analysis as a robust approach to investigate secreted proteins in cancer cells.