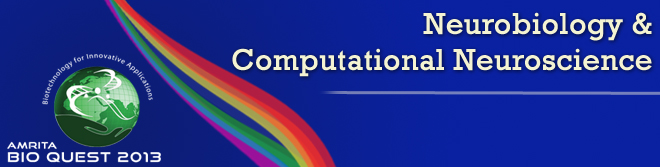
David Ibanez, Laura Dubreuil and Alejandro Rier
Neurofeedback (NF) is a type of biofeedback that uses real time display of electroencephalography to illustrate brain activity. EEG features are extracted and displayed allowing the user to, with practice, modulate their temporal evolution. Neurofeedback training has many therapeutic applications such as attention deficit hyperactivity disorder (ADHD), migraine, depression or conduct disorders. This document presents NeuroSurfer, a novel general-purpose tool for neurofeedback training with a use case of attention deficit hyperactivity disorder (ADHD) treatment.
Karmeshu, Ph.D.
Dean & Professor, School of Computer & Systems Sciences & School of Computational & Integrative Sciences, Jawaharlal Nehru University, India.
Interspike Interval Distribution of Neuronal Model with distributed delay: Emergence of unimodal, bimodal and Power law
The study of interspike interval distribution of spiking neurons is a key issue in the field of computational neuroscience. A wide range of spiking patterns display unimodal, bimodal ISI patterns including power law behavior. A challenging problem is to understand the biophysical mechanism which can generate the empirically observed patterns. A neuronal model with distributed delay (NMDD) is proposed and is formulated as an integro-stochastic differential equation which corresponds to a non-markovian process. The widely studied IF and LIF models become special cases of this model. The NMDD brings out some interesting features when excitatory rates are close to inhibitory rates rendering the drift close to zero. It is interesting that NMDD model with gamma type memory kernel can also account for bimodal ISI pattern. The mean delay of the memory kernels plays a significant role in bringing out the transition from unimodal to bimodal ISI distribution. It is interesting to note that when a collection of neurons group together and fire together, the ISI distribution exhibits power law.
Srisairam Achuthan, Ph.D.
Senior Scientific Programmer, Research Informatics Division, Department of Information Sciences, City of Hope, CA, USA
Applying Machine learning for Automated Identification of Patient Cohorts
Srisairam Achuthan, Mike Chang, Ajay Shah, Joyce Niland
Patient cohorts for a clinical study are typically identified based on specific selection criteria. In most cases considerable time and effort are spent in finding the most relevant criteria that could potentially lead to a successful study. For complex diseases, this process can be more difficult and error prone since relevant features may not be easily identifiable. Additionally, the information captured in clinical notes is in non-coded text format. Our goal is to discover patterns within the coded and non-coded fields and thereby reveal complex relationships between clinical characteristics across different patients that would be difficult to accomplish manually. Towards this, we have applied machine learning techniques such as artificial neural networks and decision trees to determine patients sharing similar characteristics from available medical records. For this proof of concept study, we used coded and non-coded (i.e., clinical notes) patient data from a clinical database. Coded clinical information such as diagnoses, labs, medications and demographics recorded within the database were pooled together with non-coded information from clinical notes including, smoking status, life style (active / inactive) status derived from clinical notes. The non-coded textual information was identified and interpreted using a Natural Language Processing (NLP) tool I2E from Linguamatics.
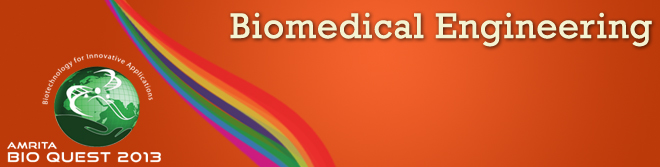
Arathy R and Binoy B Nair
PC based heart sound monitoring system
Heart diseases caused by disorders of the heart and blood vessels, are world’s largest killers. Early detection and monitoring of heart abnormalities is essential for diagnosis and effective treatment of heart diseases. Severalmethodologies are used for screening and diagnosing heart diseases. They are auscultation, electrocardiogram (ECG), echo-cardiogram, ultrasound etc. The effectiveness and applicability of all these diagnostic methods are highly dependent on the equipment cost and size as well as skill of the physician. This paper presents the design and development of a low cost portable wireless/tubeless digital stethoscope which can be used by the physician for monitoring the patient from a distance. The stethoscope system interfaces to a PC and is also capable of analyzing the heart sounds and identifying abnormalities in the heart sound and its classification. Storage of heart sound for later analysis is also possible.This advanced functionality increases the physician’s diagnostic capability, and such a PCG is not still available in most hospitals. Acoustic stethoscope can be changed into a digital stethoscope by inserting an electric capacity microphone into its diaphragm (Wang, Chen and Samjin, 2009).