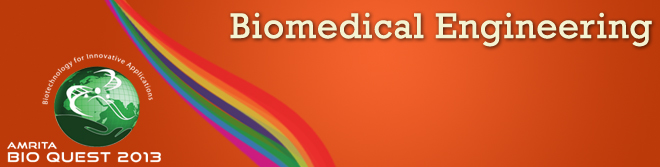
Jaydeep Unni, Ph.D.
Sr. Project Manager, Robert Bosch Healthcare Systems, Palo Alto, CA
Remote Patient Monitoring – Challenges and Opportunities
Remote Patient Monitoring (RPM) is gaining importance and acceptance with rising number of chronic disease conditions and with increase in the aging population. As instances of Heart diseases, Diabetes etc are increasing the demand for these technologies are increasing. RPM devices typically collect patient vital sign data and in some case also patient responses to health related questions. Thus collected data is then transmitted through various modalities (wireless/Bluetooth/cellular) to Hospitals/Doctor’s office for clinical evaluation. With these solutions Doctors are able to access patient’s vital data ‘any time any where’ thus enabling them to intervene on a timely and effective manner. For older adult population chronic disease management, post-acute care management and safety monitoring are areas were RPM finds application. That said, there are significant challenges in adoption of Remote Patient Monitoring including patient willingness and compliance for adoption, affordability, availability of simpler/smarter technology to mention a few. But experts contend that if implemented correctly Remote Patient Monitoring can contain healthcare expenditure by reducing avoidable hospitalization while greatly improving quality of care.
Srisairam Achuthan, Ph.D.
Senior Scientific Programmer, Research Informatics Division, Department of Information Sciences, City of Hope, CA, USA
Applying Machine learning for Automated Identification of Patient Cohorts
Srisairam Achuthan, Mike Chang, Ajay Shah, Joyce Niland
Patient cohorts for a clinical study are typically identified based on specific selection criteria. In most cases considerable time and effort are spent in finding the most relevant criteria that could potentially lead to a successful study. For complex diseases, this process can be more difficult and error prone since relevant features may not be easily identifiable. Additionally, the information captured in clinical notes is in non-coded text format. Our goal is to discover patterns within the coded and non-coded fields and thereby reveal complex relationships between clinical characteristics across different patients that would be difficult to accomplish manually. Towards this, we have applied machine learning techniques such as artificial neural networks and decision trees to determine patients sharing similar characteristics from available medical records. For this proof of concept study, we used coded and non-coded (i.e., clinical notes) patient data from a clinical database. Coded clinical information such as diagnoses, labs, medications and demographics recorded within the database were pooled together with non-coded information from clinical notes including, smoking status, life style (active / inactive) status derived from clinical notes. The non-coded textual information was identified and interpreted using a Natural Language Processing (NLP) tool I2E from Linguamatics.
Bodo Eickhoff, Ph.D.
Senior Vice-President, Head of Sales and Marketing for Roche Applied Science, Germany
New paths for treatment of complex diseases: target combinatorial drug therapy
Several types of diseases show a complex pathogenesis and require targeted as well as combinatorial drug treatment. A classical example, Tuberculosis, was thought for decades to be managable by triple therapy, however now requiring new therapeutic approaches due to multi drug resistant strains. HIV and AIDS can only be kept under control by combinations of specific, virus-protein targeted drugs, requiring constant monitoring of resistance patterns and modulation of drug combinations during life-long therapy. As a third example, Cancer in all its different variations, requires detailled molecular understanding to enable targeted therapy. New technologies provide more and in depths molecular insights into pathomechanisms and resulting treatment options. However, is there an alternative way to approach complex diseases by holistic models? Can restoring of apoptosis-capabilities of transformed cells be an example of such an alternative path? How do we in future adress major unresolved topics like increasing drug resistance in bacterial infections, lack of anti-viral drugs, treatment of parasite diseases like Malaria, and newly emerging infectious diseases in research and fast translation of these results into diagnosis and treatment?